On a rainy day in 1950, Alan Turing, the godfather of modern computing, served the world a piping hot plate of intellectual intrigue: a test designed to decipher if a machine could match wits with a human. This litmus test of artificial brain power, known as the “Imitation Game” in Turing’s era, has since donned a more relatable name, and one most readers will recognize: The Turing Test.
Its setup is about as straightforward as it gets: a human judge (Player C) has to discern between a human (Player A) and a machine (Player B) solely through written interaction. But here’s the thing—the Turing Test simply measures if a machine can mimic human intelligence, and well, not all AI is made to replicate us, is it?
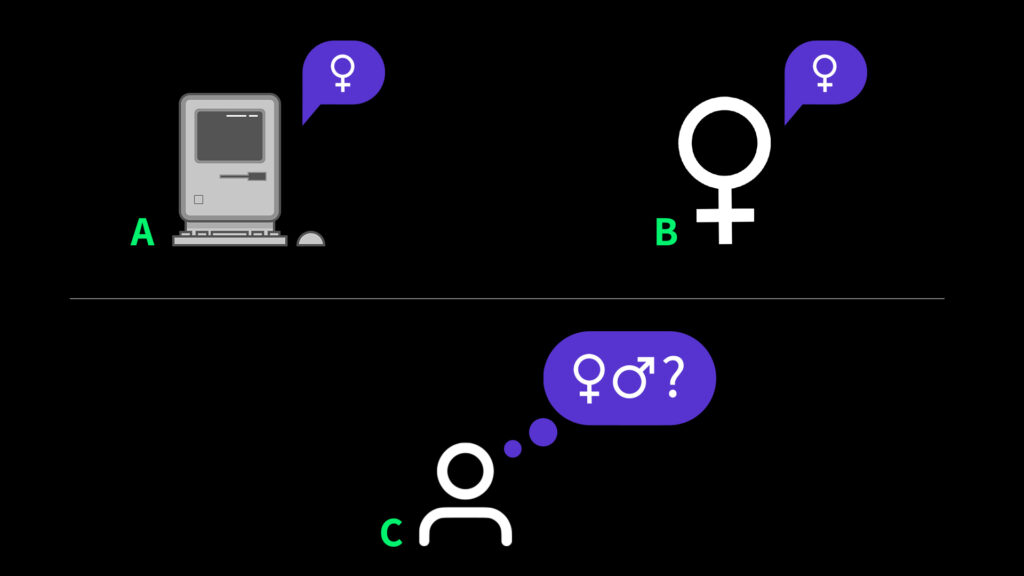
With that, let’s embark on a journey to answer three pressing questions: 1. What are the strengths and weaknesses of the Turing test in our current AI landscape? 2. What could be more suitable alternatives? 3. How should these alternatives be applied to different types of AI?
Buckle up!
Playing devil’s advocate with the Turing Test
In its heyday, the Turing test was the torchbearer for conversations on AI self-awareness, kindling debates in the realms of art and philosophy. Alas, in the sprint towards product development, our philosophical torchbearers don’t quite make the cut.
Sorry, philosophy, it’s not you, it’s us. 🤷
Despite its laurels, the Turing test falls short in its vision of AI progress. It can’t gauge how close a machine is to matching human intelligence. Think of it this way, it’s like trying to win an everlasting game of hide and seek—the machine is deemed a failure at the first slip-up, with no time limit as a cushion.
Interestingly, machines now risk detection due to their advanced intelligence, often surpassing human capabilities. So, the Turing Test creates a paradox: a machine too intelligent might fail for lack of human-like errors. After all, what use is AI that needs to be dumbed down?
More than a linguistic beauty contest, the Turing test overlooks crucial facets of human intelligence, like problem-solving, creativity, and social awareness. Howard Gardner’s Theory of Multiple Intelligences, proposed in 1983, underscores this by identifying 8 types of intelligence, reminding us that we’re more than just our chat skills.
Sorry, Turing.
Searching for the post-Turing compass
Let’s take a step into the wonderful world of alternatives to the classic Turing Test. These modern contenders have emerged to better evaluate the ever-advancing capabilities of AI. Each with a unique twist, these alternatives not only challenge AI in different ways, but also shine a spotlight on various aspects of intelligence.
The Turing Time: Given the quixotic challenge of passing the Turing test, why not measure how long a machine can convincingly imitate a human? Introducing the Turing Time: the longer the masquerade, the closer the machine is to simulating human interaction.
Lovelace Test 2.0: Up next is the Lovelace Test 2.0, which pushes an AI to create an artifact so unique that it couldn’t have been made without independent thought, thereby explaining its creation process. This test is great for gauging creativity, but falls short on assessing other aspects of intelligence.
Winograd Schema: The Winograd Schema test assesses AI’s understanding of language by posing multiple-choice questions with ambiguous pronouns. While it’s good at testing linguistic understanding, it ignores other vital facets of intelligence.
The Marcus Test: The Marcus Test challenges an AI to design a narrative with provided sentences and then explain the logic behind its choices. It excels at gauging linguistic capabilities but struggles with other aspects of intelligence.
Reverse Turing Test: Lastly, we have the Reverse Turing Test—the UNO Reverse card of AI testing. Here, a human must convince an AI judge of their humanity. It’s a great litmus test for AI judgment, but doesn’t cover the full spectrum of intelligence.
Test driving alternative AI assessments, tuned to categories
Not all AIs are cut from the same cloth. It’s about time we explored sector-specific tests.
And as we dive into the bustling world of artificial intelligence, it becomes clear that different branches of AI have their own unique challenges and, consequently, require different success yardsticks. Each AI category has a champion metric that reigns supreme, mastering in measuring the machine’s performance within that specific domain.
Let’s plunge into this topic, shall we?
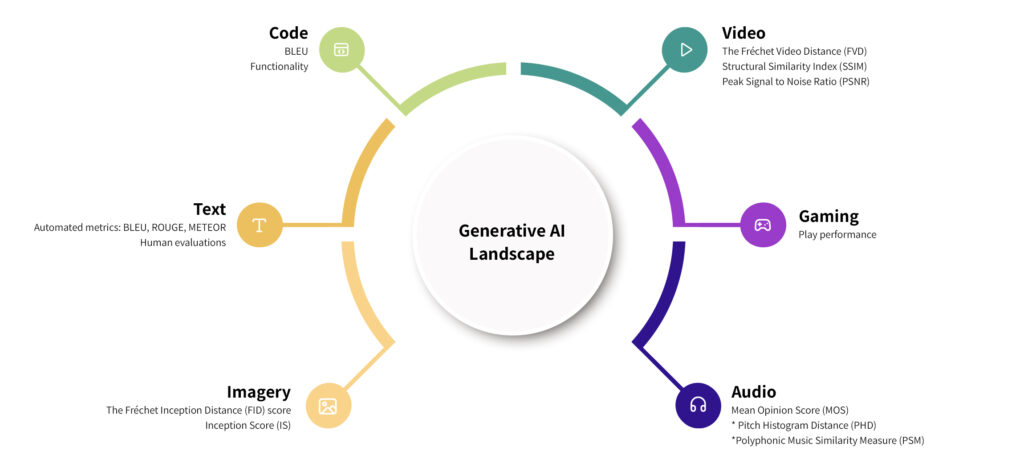
Imagery: The Fréchet Inception Distance (FID) score
For imagery, our pick is the Fréchet Inception Distance (FID) score. This resourceful method inspects the quality of AI-generated images by calculating the disparity between features in these images and their real-world counterparts. When it comes to the FID score, less is more. AKA a lower score symbolizes superior image quality and diversity. While the Inception Score (IS) might be a contender, it falls short as it neglects to juxtapose generated images with real ones.
Text: Automated metrics and human evaluations
Moving on to text, automated metrics like BLEU, ROUGE, and METEOR take the cake. These are instrumental in assessing the quality of machine-generated text against human-created reference texts. However, their application is not without limitations. Plus, their correlation with human judgment often leaves much to be desired. Hence, human evaluations, focusing on fluency, coherence, relevance, and creativity, often serve as the gold standard as well.
Code: BLEU and functionality
As for code generation, BLEU comes into play again, examining AI-generated code against human-written reference code. But in this arena, functionality is as important as syntax. Hence, the true test is not just whether the code is syntactically correct, but also if it delivers the expected functionality, for which automated testing tools can be employed.
Video: The Fréchet Video Distance (FVD)
In the realm of video, the Fréchet Video Distance (FVD) trumps. While the Structural Similarity Index (SSIM) and Peak Signal to Noise Ratio (PSNR) serve as fair tools for comparing the quality of AI-produced video frames with a reference video, they overlook temporal consistency. The FVD, with its evaluation of both spatial and temporal traits of video clips, offers a more comprehensive assessment.
Gaming: Play performance
In the field of gaming, performance is largely judged by the AI’s success rate against human players or other AI opponents. However, the proficiency of AI learning from experience and adapting to unanticipated scenarios should not be underestimated. An example would be DeepMind’s AlphaStar, which was appraised on its capacity to outplay top human contenders in the game StarCraft II.
Audio: Mean Opinion Score (MOS)
Finally, for audio, the Mean Opinion Score (MOS) is a popular pick, where human listeners rate the naturalness of AI-generated speech. For music, human evaluations are often the norm, as text metrics like BLEU don’t hit the right notes. But strides are being made with metrics like Pitch Histogram Distance (PHD) and Polyphonic Music Similarity Measure (PSM) that assess AI-generated music against human-produced music on the basis of specific musical traits.
At WellSaid Labs, we’ve adopted and excelled in these modern metrics, aiding us in achieving human parity in our AI models. Not only do these alternatives help evaluate our progress, but they also guide us in enhancing our AI systems to provide better, more human-like experiences.
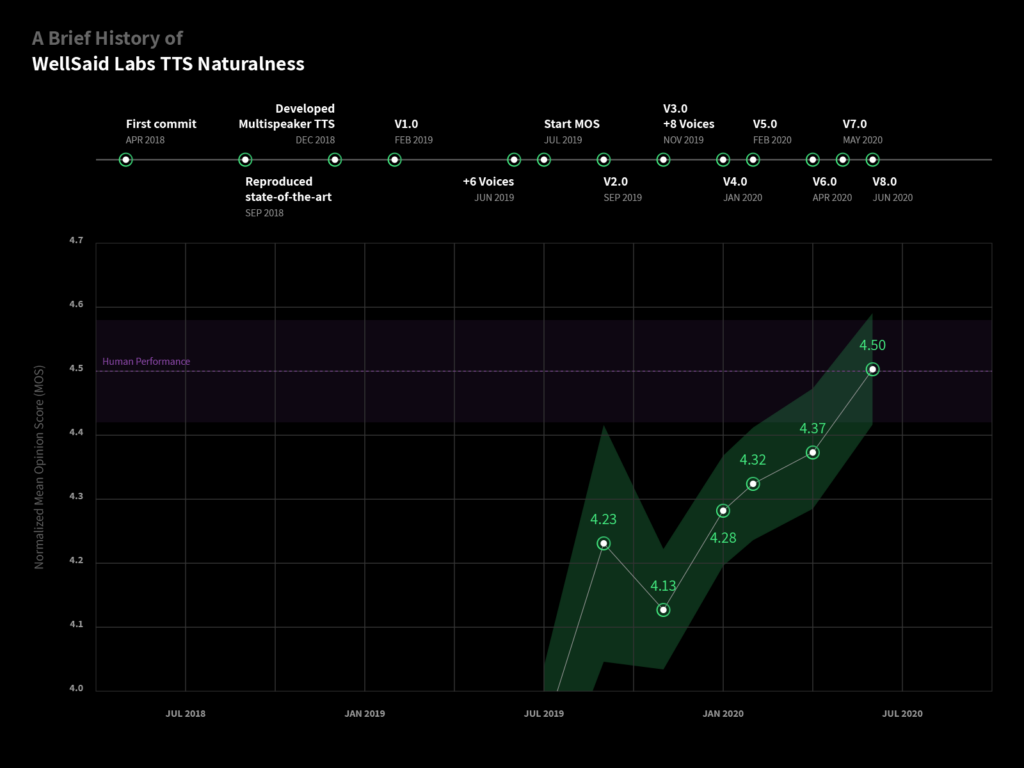
Final thoughts on AI assessments
Turing set the stage, but the play has evolved—and quite far at that. The evaluation of AI’s intelligence needs a nuanced approach, considering its diversity and purpose. While we honor Turing’s contribution, let’s venture beyond the 1950s, embracing new measures that truly capture the multifaceted brilliance of AI.
After all, isn’t it more about the journey than the destination? Stay curious, explorers.🫡